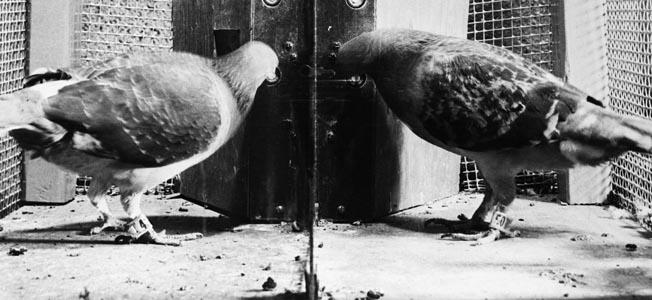
Behaviorism cast a long and wide shadow back in the day, and some of its influence can still be felt within the AI discipline. I want to focus on a few habits that are apparently left-over from behaviorist psychology, and probably detrimental to AI research. Of course I will also highlight what was right and informative about behaviorism. Behaviorism can be roughly split into methodological, radical/psychological, and analytical behaviorism. You can find more about the types of behaviorism in the main Stanford encyclopedia article, and it’s rather excellent, I recommend you to read it in entirety.
Well, basically methodological behaviorism defends an empiricist approach to study of psychology, and it is more or less the only lasting contribution of the behaviorist school. Radical behaviorism of Skinner meant that we should only be concerned with the external behavior of organisms, and reinforcements. While analytical behaviorism is even more nonsensical as it recommends us to identify mental states with behavior. Analytical behaviorism is just flat out wrong and does not need to be addressed at all. However, radical behaviorism has survived in AI literature in the form of reinforcement learning (RL), which was a central theme in Skinner’s program.
RL is in fact so influential, that some AI theorists like Dr. Hutter claim that a certain universal RL model called AIXI (which basically substitutes universal induction into the usual optimization problem of RL), is the very definition of intelligence. Are there any echoes of radical behaviorism in Hutter’s and other colleagues’ work? And if there is, are they just conceptual conventions, or is there something deeper?
There is first the definitional issue. If we claim that RL is the definition of intelligence, then that would be admitting at least part of analytical behaviorism, which is, philosophically speaking, as appealing as Plato’s theory of forms. And that’s a pretty horrid philosophical theory because it disregards what cognitive scientists might call “internal states”. It also disregards common features of brains like memory, imagination, creativity, curiosity, and so forth, and completely disregards any innate features of the brain. That is not saying that Chomsky’s critique of behaviorism was completely right, but you can find too much evidence in present day neuroscience to the contrary. Therefore, I will suggest that the convenience of analytical behaviorism must not be assumed in AI, and an RL model should not be accepted as a definition of intelligence (or psychology, intelligence is part of psychology). There are stronger philosophical arguments why not, which I will briefly mention in the passing.
What interests me are some wrong assumptions that AI researchers repeat, which seem to have been inherited from Skinner’s not-so-explanatory experiments about reinforcement learning. Skinner’s experiments essentially trivialized extremely sophisticated adaptation capabilities to reinforcement schedules, and delayed rewards (time displacements), etc. It was a somewhat unproductive kind of scientism, because it was the wrong kind of reduction, or it turned out to be. Assuming methodological behaviorism should not be an excuse to avoid neuroscience. On the contrary, we should see it as a challenge to better understand neural behavior. Here they are without further ado:
- Assumption: RL algorithms do not have to model the world, because there are shortcuts that do not need to represent the world at all. I can just work with utility surfaces. Not only is this manifestly wrong, but it is also quite misleading. In the case of some toy problems, this can work. However, in the general case it won’t. Therefore adopting this as an AGI research strategy will end in almost certain failure. In short, restricting the architectural emphasis on radical behaviorism concepts like “time displacements” or “delayed rewards” might cripple future performance of your system.
- Assumption: There is something special about pleasure and pain, or utility functions. No, there isn’t any such thing. The only thing that is special about AIXI is that it applies universal induction. All of the intelligence of AIXI owes to universal induction. Likewise, pain and pleasure are adaptations that organisms evolved to optimize the fitness of the organism. Intelligence is the capability to dynamically adapt to the environment in the medical literature. However, in AI, we are not restricted to “organisms”, that’s an unscientific vitalist obsession in the context of AI. Some AI researchers, like Solomonoff, do not find animal behavior interesting at all, and do not even model it (mainly because it is not any more intelligent than the general AI systems they model). Furthermore, AI can be a database or a search engine, and no real autonomy, or “pleasure” or any of those animal-like attributes are required in the design of such machines, and they can absolutely be intelligent while lacking animal-like agent architecture. AI is not identical to AI agent.
- Assumption: All agents are based on, or naturally reduced to RL agents. No, of course not. There are many ways to formulate agents, and they do not need to follow optimizing a single utility function. Like humans, there can be many motivation systems they use in combination, and complex cognitive architectures with many kinds of learning (not just RL) might happen. In particular, neuroscience suggests us that there are many basic kinds of learning. Likewise, inductive inference theory suggests to us that at the root there are non-agent-based universal machine learning algorithms (that have nothing to do with environments, rewards, or other behaviorist concepts).
- Assumption: RL is the only way to model active inference and active inference does something that passive inference cannot, it is more intelligent. These are all confused ideas, but often repeated together. Nothing in theory that I know suggests that active inference is more intelligent by virtue of basic machine learning method. Or that the only way to model active inference is RL. However, it is true that an autonomous active agent can learn more than a passive agent, because it will have access to more data (!). That is interesting, but also trivial to know.
There are also some pretty nice aspects of behaviorism that I think AI researchers took advantage of. There certainly were some lessons learnt. In no order:
- Maximizing expected utility in the long run is a good way to specify a holistic goal for an agent.
- The importance of the environment, we do owe to the behaviorist school.
- The idea that every interesting behavior can be learnt, is also somewhat attributable to the movement. In other words, a blank slate AI agent can work.
- I think the idea of applying Wittgenstein’s “meaning is use” theory of language would also come from behaviorists, and that theory is, of course, basically right. Wittgenstein may be considered as the philosophical forefather of behaviorism.
On the other hand, models like AIXI actually disprove the main philosophical claims of analytic and radical behaviorists, because within it, the AIXI model does a lot of simulations to predict the world. That means that it is imagining things. Behaviorists didn’t think that the brain does computations, or imagines things not in the environment, or simulates things, or that sort of thing, so models like AIXI run directly counter to the main tenets of radical behaviorism.